Artificial intelligence in special education
AI • Mar 21,2017
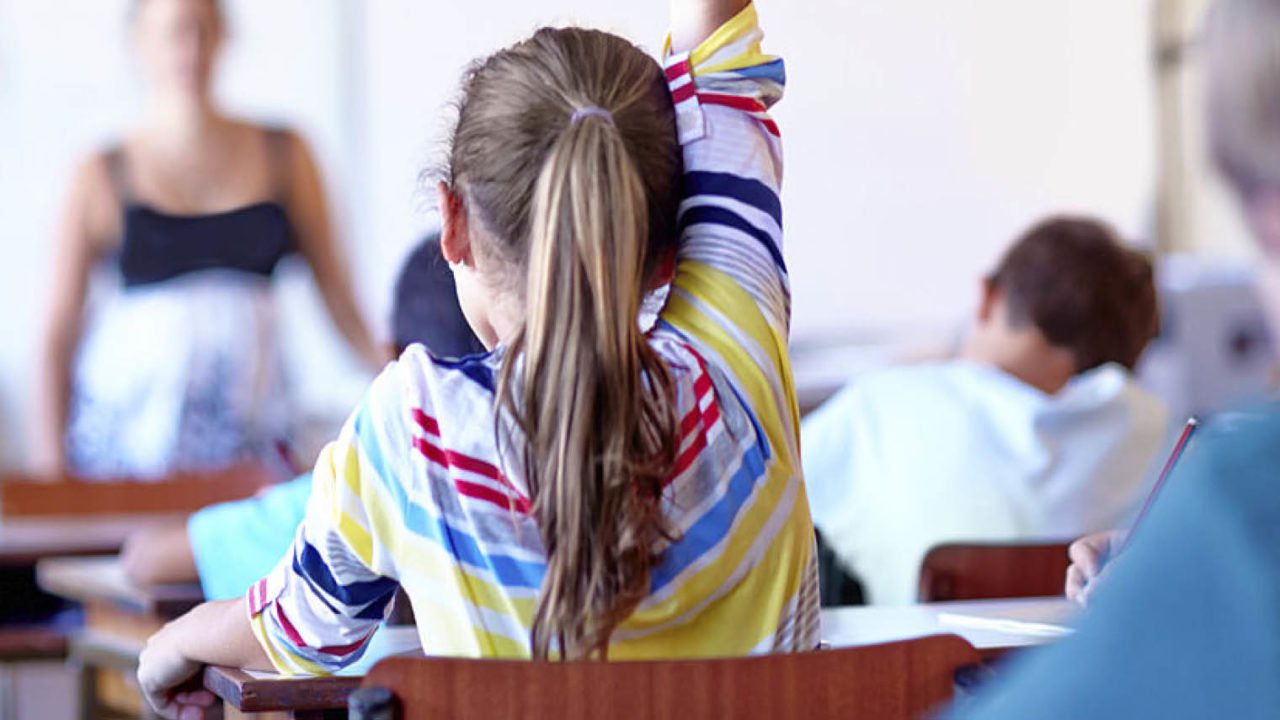
Artificial intelligence in special education
Artificial intelligence technologies have started to open new ways of interacting with students with special educational needs. The goal of these tools is to enhance the way children interact with their environment to promote learning and to enrich their daily life. The effective applications of AI techniques is seen as a means of improving the quality of life of students with special education needs.
The term ‘special educational needs’ refers to a wide range of difficulties which cause problems in learning. Identifying individual’s weaknesses in different areas as well as establishing correct diagnosis and educational plan had been always a difficult area as some disabilities are more settled and with possibly some other disabilities and disorders overpowering and interfering capabilities of individual that may appear to have certain learning disabilities.
Link-up between artificial intelligence educational approaches and students with different abilities and needs has opened new eras and trends which require use of specific tools and new methods in order to improve children’s life in both school and home settings. During last decade a big number of studies, which addresses use of AI techniques in special education, was published.
In 2003 Georgopoulos presented a fuzzy cognitive map approach for differential diagnosis of specific language impairment (SLI). Fuzzy cognitive maps are a soft computing methodology that uses a symbolic representation for the description and modeling of complex systems. The aim of this tool is to provide the specialists with a differential diagnosis of SLI from dyslexia and autism, since in many cases SLI is difficult to be discerned due to its similar symptoms to other disorders. The system has been tested on four clinical cases with promising results.
In 2008 Arthi and Tamilarasi introduced a model which helps in the diagnosis of autism in children by applying artificial neural networks technique. The model converts the original autistic data into suitable fuzzy membership values and these are given as input to the neural network architecture. Moreover, a pseudo algorithm is created for applying back propagation algorithm in predicting the autistic disorder. This approach is proposed to support apart from medical practitioners, psychologists and special educators. In future, the autistic disorder could be predicted using k-nearest neighbor algorithm for a comparative research
Another great example of AI in special education is a research by Jain. He proposed a model called perceptron based learning disability detector (PLEDDOR). It is an artificial neural network model for identifying difficulties in reading (dyslexia), in writing (dysgraphia) and in mathematics (dyscalculia) using curriculum based test conducted by special educators. This computational diagnostic tool consists of a single input layer with eleven units that correspond to different sections of a conventional test and one output unit. The system was tested on 240 children collected from schools and hospitals in India and was evaluated as simple and easy to replicate in huge volumes, but provides comparable results based on accepted detection measures. Kohli introduced a systematic approach for identification of dyslexia at an early stage by using artificial neural networks. This approach is amongst the first attempts which have been made for addressing the dyslexia identification problems with the use of ANN.
As we may see artificial intelligence techniques have successfully been applied to solve problems in the field of special education. Scientists believe that with help of AI students can move toward personal learning goals. The identification of students with special abilities and learning difficulties can provide a valid and accurate diagnosis which later will help to choose the most appropriate intervention method.
Author: AI.Business
If you like our articles, please subscribe to our monthly newsletter:
[mc4wp_form id=”763″]
Previos Article Predictive analytics as a future of higher education
Next Article Machine learning and energy efficient building design