How AI can help identify weeds and increase field productivity and profitability
AI • Dec 21,2016
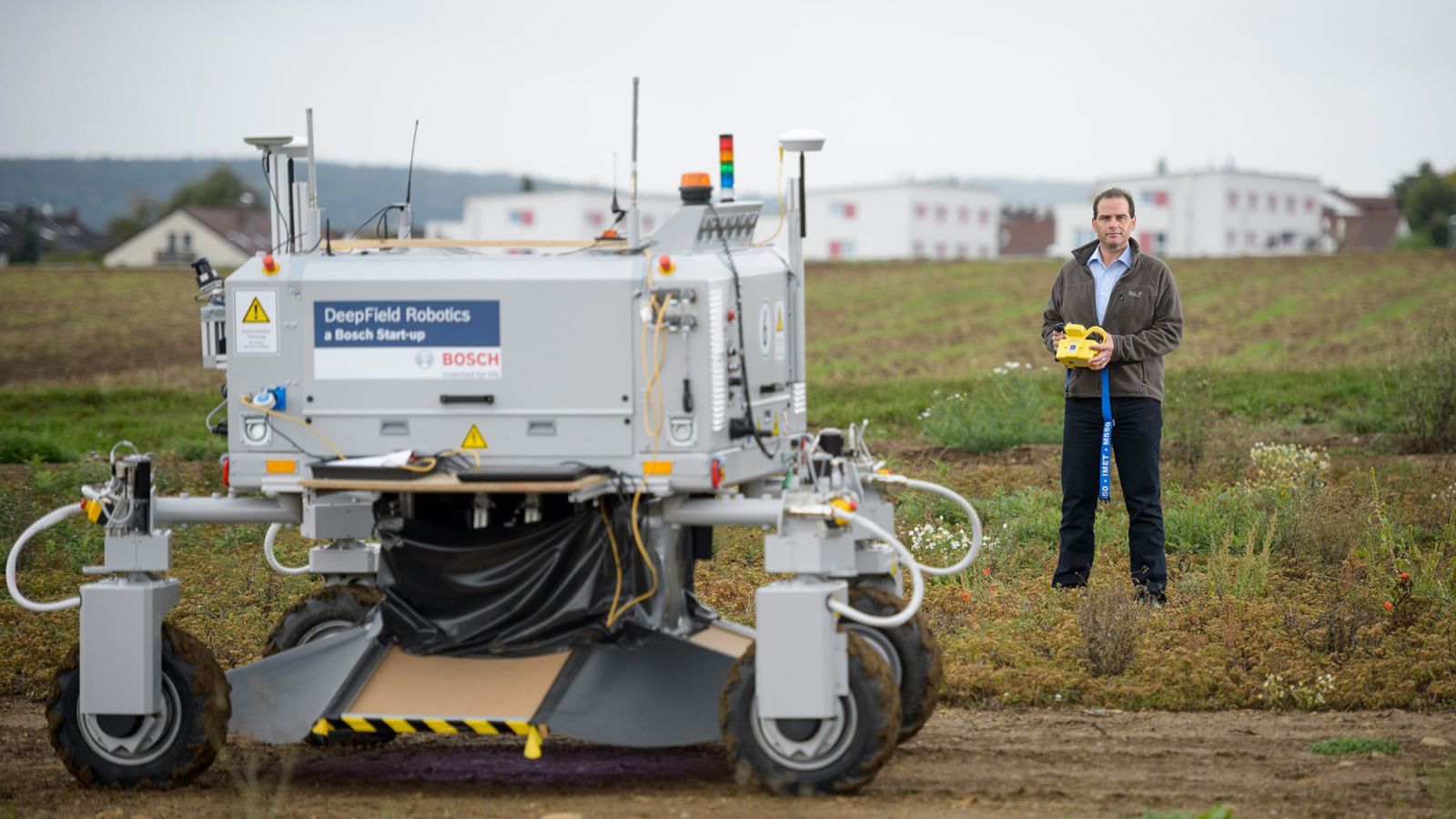
How AI can help identify weeds and increase field productivity and profitability
In a world reached a population of six billion humans increasingly demand it for food, feed with a water shortage and the decline of agricultural land and the deterioration of the climate needs 1.5 billion hectares of agricultural land and in case of failure to combat pests needs about 4 billion hectares. Weeds represent 34% of the whole pests while insects, diseases and the deterioration of agricultural land present the remaining percentage. Weeds identification has been one of the most interesting classification problems for Artificial intelligence and image processing.
The most common case is to identify weeds within the field as they reduce the productivity and harm the existing crops. Success in this area results in an increased productivity, profitability and at the same time decreases the cost of operation. When AI algorithms combined with appropriate imagery tools may present the right solution to the weed identification problem.
Weeds are unwanted plants that reduce available moisture, nutrients, sunlight and growing space needed by crop plants. Their presence can reduce crop growth, quality and yielding. In addition, they can make harvest difficult. Weeds also provide cover for diseases, insects and animals (rodents, box turtles, snakes). There are several methods that should be combined and a lot of efforts should be coordinated to control weeds including cultural, mechanical and chemical methods.
Herbicides are another weed control aid that some specialists employ. Increased use of herbicides in the crop and non- crop areas, such as hedgerows, results in dramatic reduction in numbers and types of weeds. However, this causes an even greater reduction in the number of species and populations of beneficial insects (those that kill crop pests). This resulted in an increase in pest populations, requiring more pesticides to be used, resulting in increased costs.
Modern technologies of cultivated crop growth during plant growing, which use chemical compositions to avoid weeding, lead to pollution of the environment and products of plant growing with these compositions leading to decayed production. Moreover, purchasing weed killers considerably increases the costs of the crop cultivation.
Making every plant count is at the heart of Blue River Technology’s mission. The California-based company has developed a pioneering approach that utilizes computer vision and robotics to build a solution where the needs of each plant are precisely measured and delivered while significantly reducing chemical use.
“We want to be able to recognize weeds and spray just the weeds and not the crops,” explains cofounder Jorge Heraud. “This has several advantages. It doesn’t require selective herbicides, as selectivity is provided by our machine. This opens the door to new chemistries and allows us to move from agriculture’s overreliance on herbicides. For example, Roundup represents close to 90% of all herbicide used in the U.S. However, it’s not effective on approximately 60,000,000 acres due to overuse.”
He says the company’s machine, which was developed with partner Lee Redden, can also reduce chemical use by up to 10 times because it only targets weeds. “This would not only save in chemical costs, but also reduce the environmental impact,” explains Heraud. “This technology could even bypass the need for genetic engineering of herbicide-tolerant plants.”
The duo’s vision is rapidly becoming a reality with LettuceBot. “Through a thinning process, our machine is capable of measuring and spraying 5,000 plants per minute,” says Heraud.
Venkatesh and Thangaraj (2008) applied Artificial Intelligence (AI) techniques to find the best match of crop(s) for the given type of soil characteristics. AI was used for weeds identification using an evolutionary artificial neural network to minimize the time of classification training and minimize the error through the optimization of the neuron parameters by means of a genetic algorithm.
They presented evolutionary self- organizing map neural network and demonstrated its capabilities to recognize and classify image patterns that represents different planet leaves including weeds. The intent of the evolution process is the maximization of the neural network classification performance. The network evolution uses the basic strategy of GAs for evaluating and recombining the fittest input parameters as spectral feature reference values.
The strategy is repeated over two phases: An evaluation phase and a reproduction phase. During the evaluation phase, leaf optical features are evaluated based on the performance of the network in which they participate. The obtained results indicate that GA, with its global search capability, finds the optimum features. These evolved features enhanced the performance of the classification system.
Since neural network classifiers are well suited for real-time control applications, the achieved results suggest that vision systems can achieve real-time discrimination of weed so hardware and software design must be conducted to develop an integrated real-time image processing and an evolutionary neural network classification system to be used directly in the field for real time classification and control for the fertilization machines.
Author: AI.Business
If you like our articles, please subscribe to our monthly newsletter:
[mc4wp_form id=”763″]
Previos Article Identifying Animal Growth Using Artificial Intelligence
Next Article How AI is changing Asian agriculture industry