Predictive analytics as a future of higher education
AI • Mar 15,2017
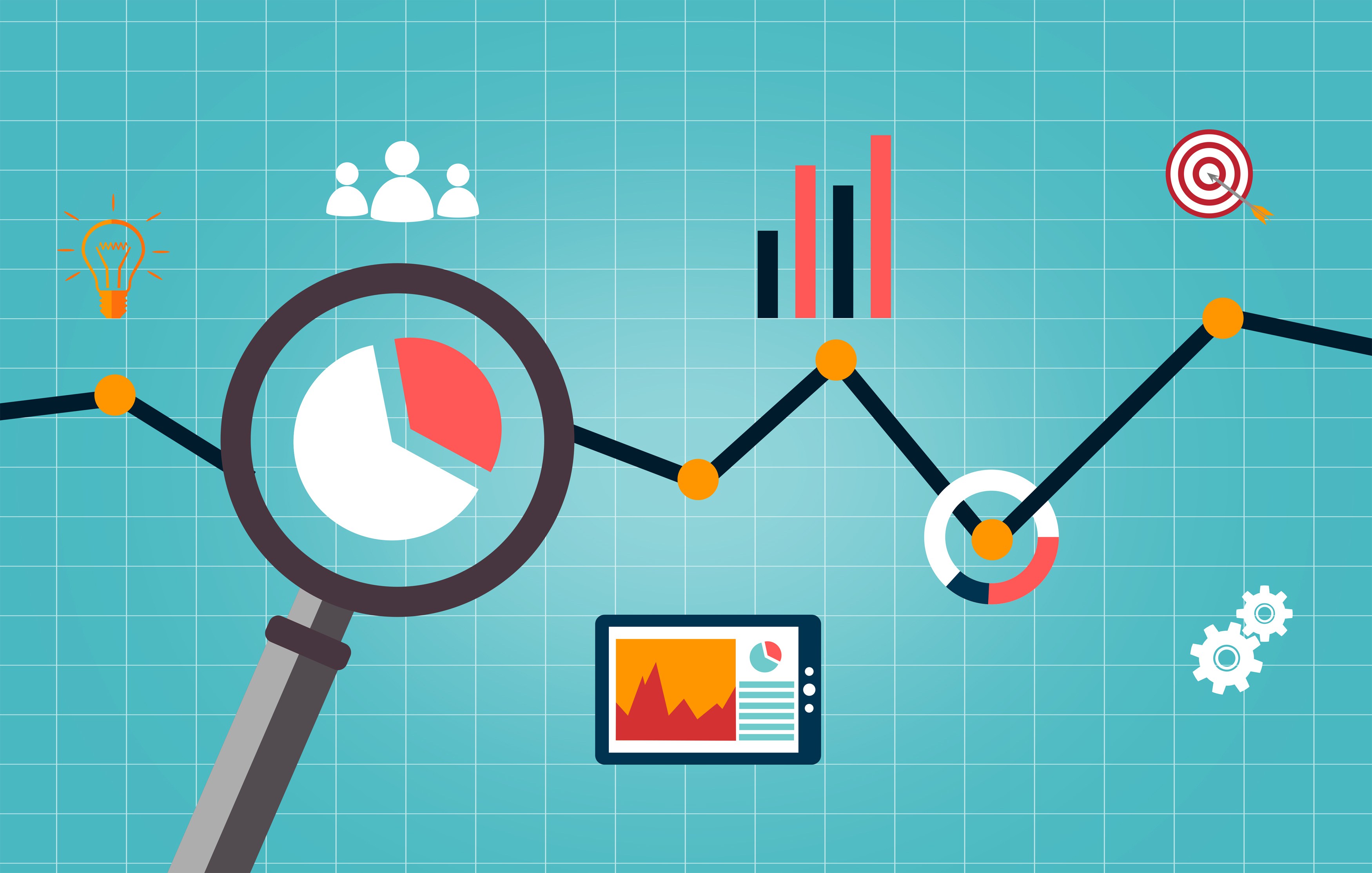
Predictive analytics as a future of higher education
There’s a lot of focus on digital transformation initiatives in the learning technology space over the past few years. Now higher education leaders are starting to realize what technology can do for their institutions. From determining which students are most at risk of dropping out, to supporting retention strategies to persist toward degree completion it also has the ability to improve operational efficiency and identify opportunities for growth. Predictive analytics, big data and artificial intelligence can significantly increase the odds for a higher education institution that it can deliver its own goals in an optimal manner.
Today colleges and universities are getting and using an enormous amount of data. For instance, data is being collected on students—not only regarding how they perform on a given course but regarding all aspects of student life: housing, finance, social activities (e.g., participation in student organizations, attendance at sporting events, gym membership). In addition, data is being collected on the research performance/productivity of faculty: the number of grant applications submitted, research awards received, publications and patents produced.
And finally, data is generated from institutional general operations (data on buildings, energy systems, human resources). Historically, colleges and universities have used this data for simple transaction-processing purposes such as invoicing, resource-allocation decisions, and budgeting. However, they are becoming much more sophisticated in their usage of data through predictive analytics.
Predictive analytics in higher education is a hot button topic among educators and administrators as institutions strive to better serve students by becoming more data-informed. While adoption is not easy, some colleges are using predictive analytics for multiple purposes and for various phases of a student’s academic career. Georgia State, for example, describes its GPS system as being both an early-alert system and a major matcher, which informs students of their chances of succeeding in each major.
The university is also using adaptive learning software in its hybrid introductory math courses. Adaptive learning courseware are digital tools that are responsive to students’ individual learning needs. Similarly, the historically black Delaware State University in Dover, Delaware uses predictive analytics to meet enrollment goals, identify struggling students, and streamline its advising practices.
Universities use predictive analytics for developing adaptive learning courses, which modify based on the interactions of the student with the technology. Adaptive learning has been with us for a while, with its history of adaptive learning rooted in cognitive psychology, beginning with the work of behaviorist B.F. Skinner in the 1950s, and continuing through the artificial intelligence movement of the 1970s. For example, adaptive learning technology is used by NASA for simulation training, safety models, and has been used in various branches of the U.S. military, including the Army Learning Concept 2015, that trains and educates soldiers for asymmetric warfare. Adaptive learning’s greatest potential to help the greatest number of people is in K-12 and higher education.
Here’s an another example, where Colorado Technical University partnered with Realizeit, an adaptive learning company, to develop intellipath. Intellipath uses custom content created by CTU faculty and Realizeit’s Adaptive Intelligence Engine that aims to adapt to the needs of each learner. Realizeit’s Adaptive Intelligence Engine, relies on machine learning to under and the unique abilities of students and how they process information based on how they use the tool.
The U.S. Department of Education has a national technology plan that endorses the use of technology, and adaptive learning in particular, for its ability to simultaneously teach and assess for continuous improvement. Advances in adaptive learning systems and platforms with their powerful feedback loops are used in blended learning environments for greater personalization. The ability for students to track their own learning means that they can develop valuable self-monitoring skills, and engage in their personal learning progress.
Predictive analytics is also used for targeted-student advising and increasing enrollment yield. It can help and improve the quality of education by analyzing the historical data of the student and allow the decision makers address different factors in future.
Author: AI.Business
If you like our articles, please subscribe to our monthly newsletter:
[mc4wp_form id=”763″]