The role of machine learning in construction safety
AI • Mar 29,2017
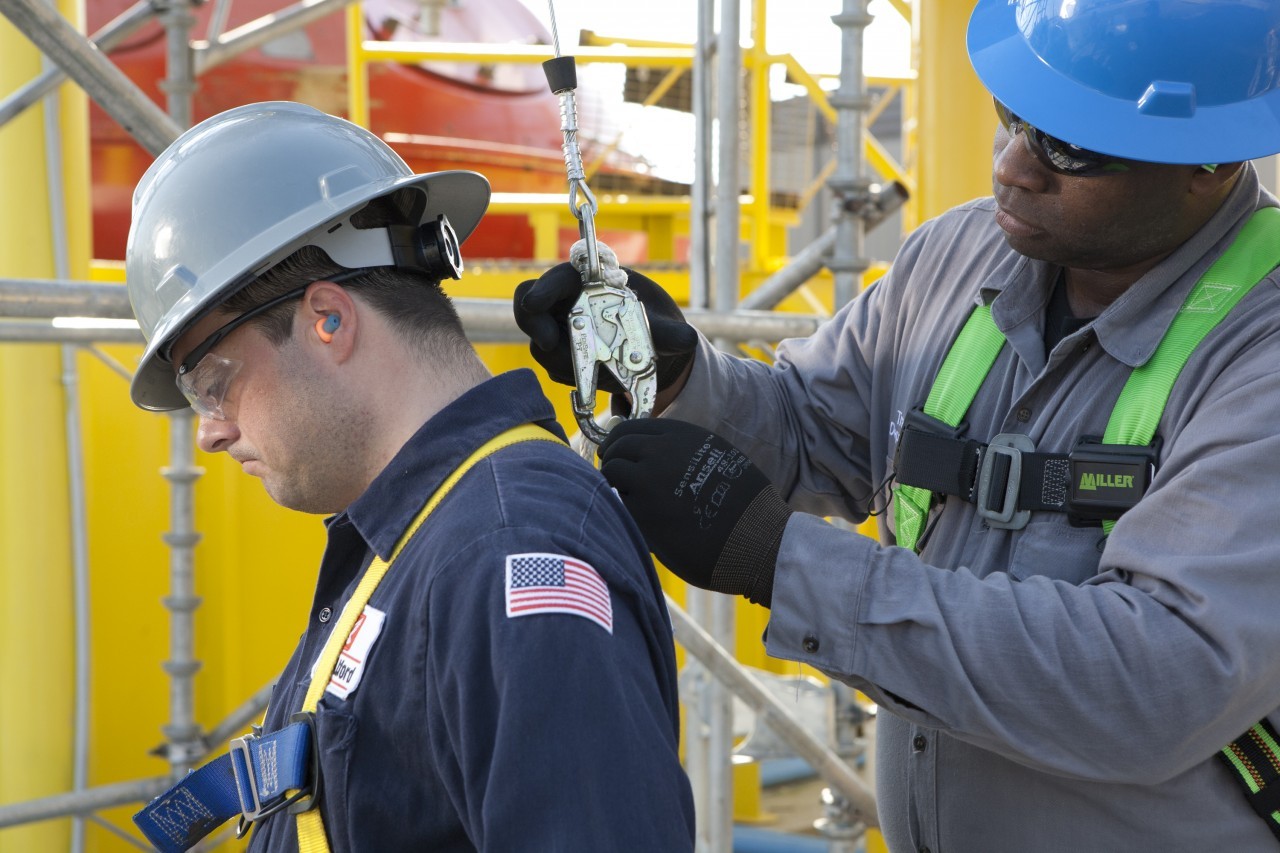
The role of machine learning in construction safety
The construction industry is one of the biggest in the USA but also is one of the deadliest. Construction has been and continues to be a dangerous occupation, resulting in many accidents, injuries and fatalities. 937 workers died on sites in 2015, meaning that one in five worker deaths last year were in construction. Safe practices are crucial for the industry. Developments of methodologies for machine learning use in construction safety and the preparation of appropriate software will fill a large need in the industry.
Analysis of construction equipment activities with a proper level of detail can help improve several aspects of construction engineering and management such as productivity assessment, safety management, idle time reduction, emission monitoring and control etc. In most cases, however, manual observation of activities is very difficult, time-consuming, and prone to error. Therefore, some automated field data collection techniques have been investigated as potential solutions to this problem. For example, simulation models used for decision-making in construction equipment activities can in particular benefit from realistic input data collected from field activities.
Risk analysis has emerged as a promising alternative to managerial and regulation-based approaches. However, construction safety risk analyses are currently limited because existing techniques overlook the complex and dynamic nature of construction sites and are not based on empirical data. Traditional construction safety research is limited as it was built on the assumption of independence of tasks and is primarily based upon expert opinion or subjective, aggregated, or secondary data.
For example, Esmaeili and Hallowell laid the groundwork of a new conceptual framework, offering a systematic and comprehensive way to extract safety critical structured information from unstructured injury reports. Unlike traditional safety risk analysis techniques, this attribute-based approach renders construction injuries as the resulting outcome of the joint presence of a worker and the interplay among a finite set of universal descriptors of the work environment that are observable before an injury occurs. These binary attributes, also called injury precursors, make physical sense and are related to construction means and methods, human behavior, and environmental conditions.
The recourse to an extended list of attributes validated by past research and to a highly accurate NLP system allowed a large data set of 4400 attributes and safety outcomes to be constituted. Then Antoine J.-P. Tixier, Matthew R. Hallowell and other researchers applied two state-of-the art machine learning algorithms, random forest and stochastic gradient tree boosting, to this structured data set. Using binary fundamental construction attributes as input, the resulting models predict three safety outcomes out of four with high skill, namely injury type, energy type, and body part. This clearly outperforms the models developed in past research in terms of skill (276% relative improvement) but also in terms of variety of outcomes predicted.
The Smartvid.io created a machine learning engine, nicknamed VINNIE (Very Intelligent Neural Network for Insight and Evaluation’), then uses speech and image recognition to analyze and organize project files. For the 2016 ENR Photo Competition, Smartvid.io partnered with ENR’s editorial staff to hold the first-ever artificial intelligence for construction safety demonstration.
VINNIE automatically scanned photos to for the presence of human workers. Simply knowing that people exist in an image is a great way to flag content a human safety expert needs to review. Next, the people-containing images were automatically checked for two specific categories of safety risks: 1) workers not wearing hard hats and/or 2) working not wearing safety vests. The algorithm was able to sort through all 1,080 submissions in under 10 minutes, while the human team required over 4.5 hours. VINNIE correctly detected 446 images with people, while the human review only found 414.
Machine learning should be given serious consideration as a technology which can be useful in construction safety. Considering its expected benefits, saved human lives and construction costs, the challenge of machine learning In construction safety should be met through continued research.
Author: AI.Business
If you like our articles, please subscribe to our monthly newsletter:
[mc4wp_form id=”763″]
Previos Article Enhancing teaching and learning through educational data mining
Next Article How IoT and AI is helping to fight crime