AI-based Solution to Identify Fraudulent Money Transfers
AI • Mar 26,2024
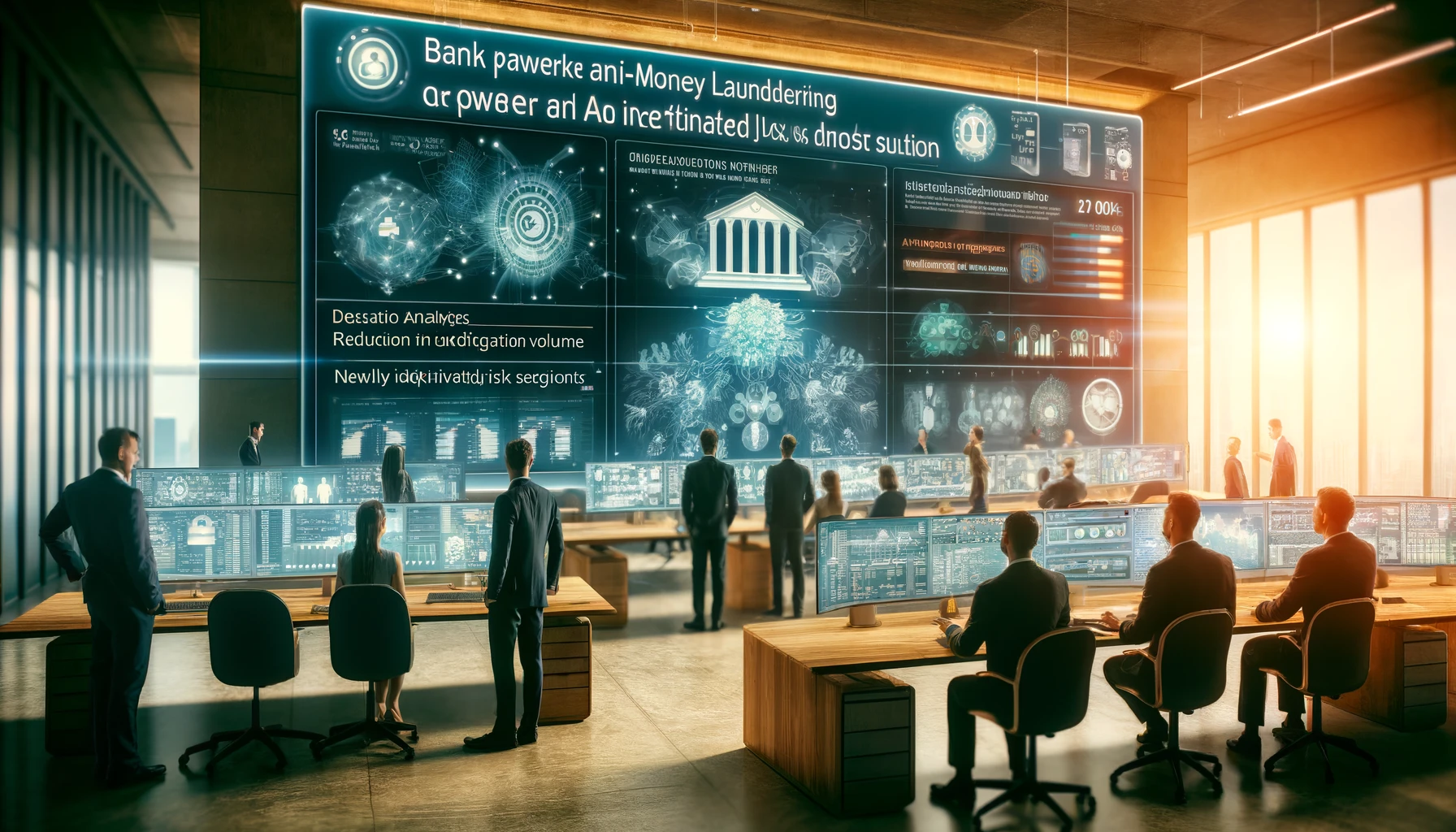
Employing an AI-powered anti-money laundering solution, the bank managed to reduce its investigative volume by over 20% and mitigate regulatory risks by identifying previously unrecognized risk segments.
Client:
HSBC is a global banking and financial services company based in London, UK. Established in 1865, HSBC boasts a long-standing history and stands as one of the largest organizations in the banking and financial services sector worldwide.
Problem Statement:
Recognizing the need for increased efficiency and more targeted detection of potential criminal activities, HSBC acknowledged the necessity of automating anti-money laundering investigations. Traditionally, these investigations were carried out manually by thousands of workers tasked with analyzing data and devising new rules—an approach that proved unsustainable. HSBC implemented numerous rules to filter transactions, but these rules lacked the flexibility and adaptability needed to effectively address money laundering threats. Standard industry practices for transaction monitoring and other forms of financial crime risk detection were largely ineffective, even when meeting regulatory requirements. HSBC set out to enhance operational efficiency in the anti-money laundering domain by 3%, with an ambitious target of 5%.
Results:
- 20% decrease in false positives, which refers to cases where the bank’s existing rules would have flagged for money laundering risk when, in reality, there was no such risk.
- A lowering of regulatory exposure by discovering new risk segments that had previously gone unnoticed.
AI Solution Overview:
HSBC collaborated with Ayasdi, a machine learning software company, to develop an AI-driven solution for combating money laundering. This software, jointly created by HSBC’s internal IT team and Ayasdi’s data scientists, is designed to recognize suspicious patterns in historical data associated with money laundering. When presented with current payment data, it can swiftly identify fraudulent patterns and notify staff to block such payments. The software scrutinizes various factors including the source and destination of payments to detect any anomalies in behavior.
HSBC’s IT experts assisted Ayasdi in comprehending the bank’s internal anti-money laundering data, while HSBC’s modeling team aided Ayasdi in crafting precise customer behavior models. This collaboration facilitated seamless utilization and integration of Ayasdi’s models into HSBC’s operational practices, thereby overcoming the typical challenge of incorporating a vendor product.
Ayasdi improved its capability to incorporate and generate features such as transactional data (type, direction, value), customer data (geographical, chronological), and risk data. Using a subset of this data, Ayasdi developed a series of potential segments. Importantly, Ayasdi did not increase the number of groups; instead, it created more intelligent, defensible, and consistent groups using entirely different features compared to those used by the bank. Remarkably, this process was conducted without supervision; Ayasdi’s software autonomously selected suitable algorithms, generated candidate groups, and fine-tuned scenario thresholds until optimal results were achieved.
The presentation and organization of these results is done within the Ayasdi platform. Ayasdi generates a decision tree to illustrate how decisions are made within the system, aiming to mitigate the “black box” issues commonly associated with machine learning, which are unacceptable in the tightly regulated finance sector. The distribution of customers across these groups was subsequently assessed, independently validated, and integrated into the bank’s existing infrastructure.
Ayasdi’s expertise extends beyond anti-money laundering to AI methodologies and result clustering. The analysis requires the specialized skills and knowledge of the HSBC teams, who evaluate the significance and utility of Ayasdi’s clusters. This enables them to strategically determine which of these distinct patterns should be adopted to update their anti-money laundering rules and systems.
References:
- Jon Asprey (2023) AI-powered compliance: Transforming banking processes for efficiency and accuracy. https://www.coreconsultants.io/resources/blog/ai-powered-compliance–transforming-banking-processes-for-efficiency-and-accuracy/
- Artificial Intelligence for Anti-Money Laundering – An Analysis of Solution. https://emerj.com/ai-sector-overviews/ai-anti-money-laundering/
- Artificial Intelligence for Risk Reduction in Banking: Current Uses. https://www.linkedin.com/pulse/artificial-intelligence-risk-reduction-banking-current-shroff/
- Bank Reduces Money-Laundering Investigation Effort with AI. https://emerj.com/ai-case-studies/bank-reduces-money-laundering-investigation-effort-with-ai/
- Anti-Money Laundering Solution Deep Dive. WHITE PAPER. https://s3.amazonaws.com/cdn.ayasdi.com/wp-content/uploads/2018/04/22170635/AML_Solutions_Deep_Dive_WP_051617v01.pdf
Vendor: Ayasdi
Client: HSBC Bank
Industry: Finance
Publication Date: 2018
Key Words: anti-money laundering, money transfers, compliance, fraud, AI-based solution, financial crimes
Previos Article AI Tool Assists Legal Workflow
Next Article 95% Fewer False Alarms: JPMorgan Chase Uses AI to Sharpen Anti-Money Laundering Efforts