ML Cuts Ferroalloy Costs 3-5% in Steel Smelting
AI • Apr 09,2024
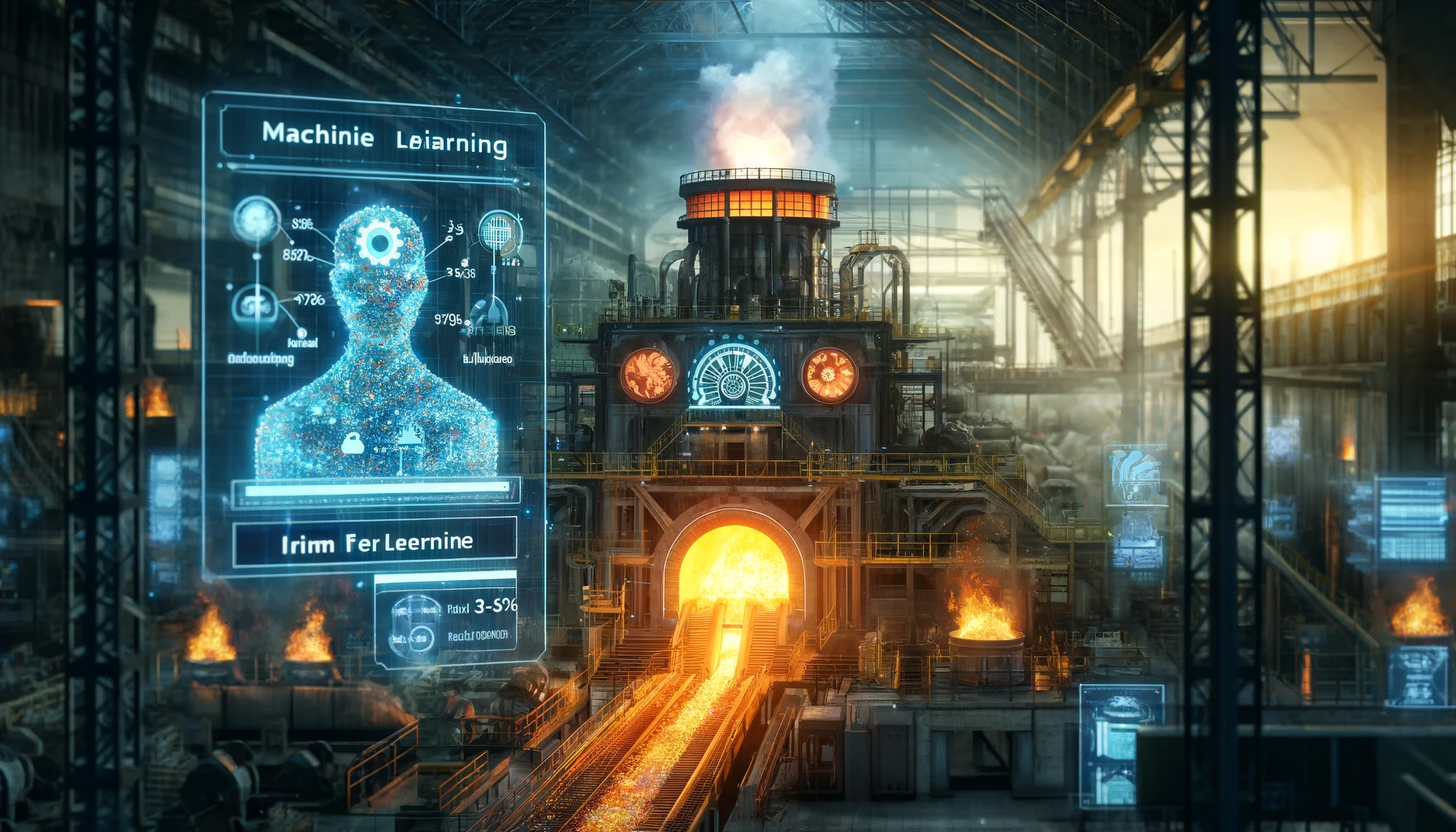
A steel manufacturer faced challenges balancing production speed, material conservation, and precise chemical composition control during smelting. They implemented an AI solution that predicts steel composition and recommends optimal additive amounts to address this. This resulted in a 3-5% reduction in expensive ferroalloy consumption, faster processing times, and stabilized product quality.
Problem Statement:
The steel manufacturer is actively involved in predicting the chemical element content within the melt composition during production. This relies on a multitude of factors, with calculations performed in real-time to adhere to restrictions and stay within specified requirements. The primary goal is to ensure compliance with chemical composition standards. Moreover, the melting process is time-constrained—the faster the process, the better. Alongside process speed, there’s a strong incentive for the steelmaker to conserve raw materials whenever feasible. Decision-making time is limited, and it’s challenging to manually keep track of all requirements for the current steel grade.
Results:
- Easier to manage production processes.
- Reduction in consumption of expensive ferrous materials, smelting time, stabilization of the chemical composition of products
- Increased processing productivity.
- Reduction of the cost of ferroalloys consumption for smelting can reach 3-5%.
AI Solution Overview:
The ML solution aids in predicting the steel composition based on decisions made by the steelmaker. It offers recommendations on the quantity of materials to add, calculating the necessary minimum to meet requirements while conserving materials efficiently. Developed for a steel smelting plant, the service calculates the minimum required proportions of additives, considering all relevant process parameters. The process of creating ML models involves several stages. Initially, data is gathered from actual production sources like process control systems and sensors, then processed and prepared. Models are subsequently built based on this prepared data, grounded in the physics of the process itself.
While physicochemical models are highly accurate, they may lack robustness concerning input data. This drawback can be addressed by employing ML models, which can operate independently of the physical model, ideally complementing and refining the real process model.
References:
- Machine Learning For Steel Smelting | AI Case Study Hub #2. https://www.linkedin.com/pulse/machine-learning-steel-smelting-ai-case-study-hub-2-dmitry-gavryukhin/
Industry: Metallurgy
Previos Article AI Improves Construction Progress Tracking and Transparency
Next Article AI-Powered Automation for Rail Edge Trimming