AI Retail Marketing: 80% Faster, $300M Saved
A I • Jun 23,2024
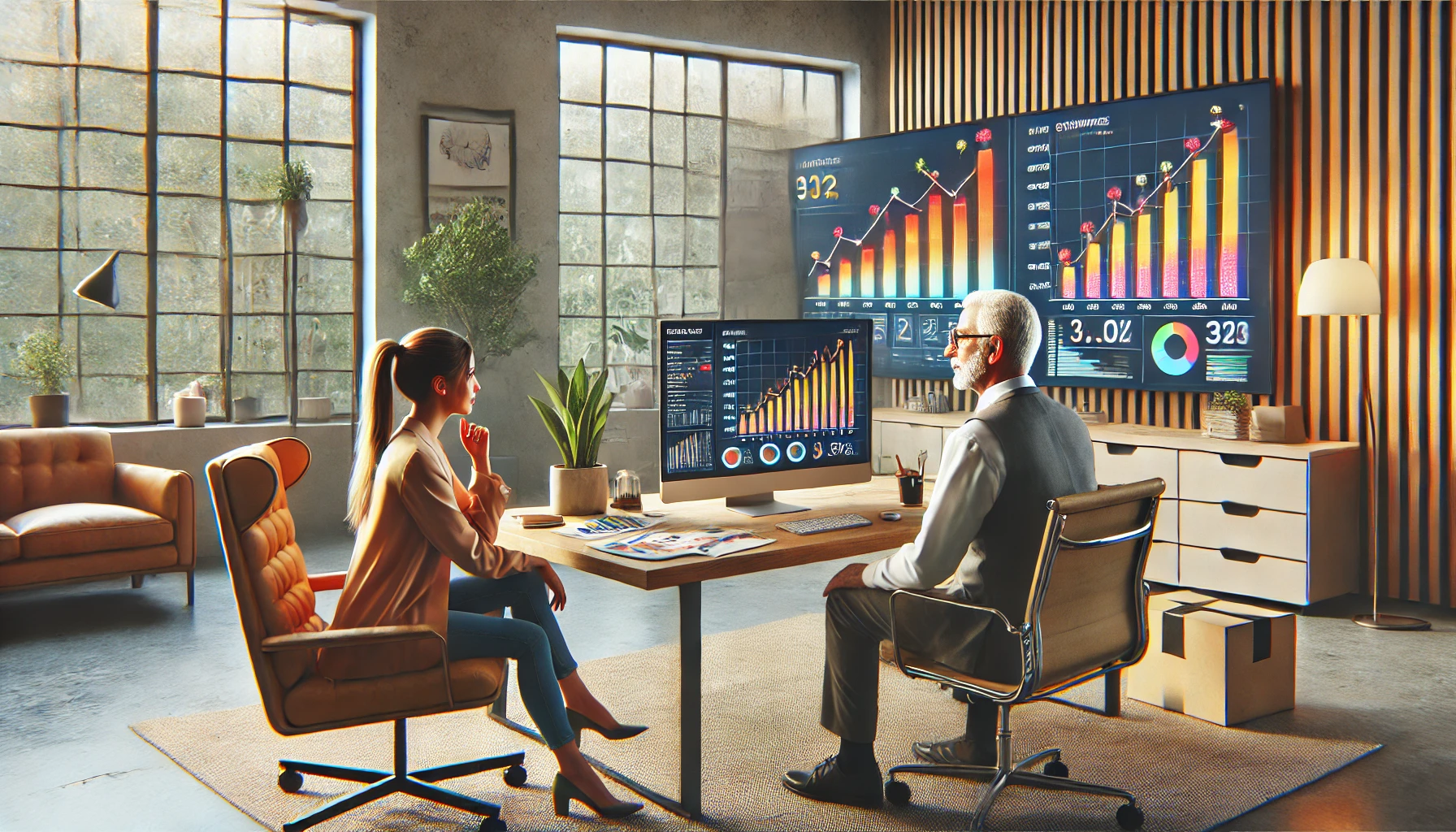
A leading US retailer faced sluggish data, limited channel understanding, and slow processes hindering marketing agility. They implemented an AI solution with machine learning for faster data insights, optimized media spending, and targeted resource allocation. This resulted in a significant boost: 80% faster data processing, better ROI, and $300 million in new media buying opportunities.
Problem Statement:
A leading US retailer grappled with the following marketing challenges:
Slow and outdated data: The retailer relied on historical data to make media spending decisions, which was often stale when it was available for analysis.
● Limited channel insights: Traditional models could not capture the complex interdependencies between different marketing channels, making optimizing media spending across multiple channels difficult.
● Lack of agility: The retailer’s existing processes were slow and manual, making it difficult to respond swiftly to customer trends and market shifts.
● Inefficient media spending: The retailer struggled to identify the most effective media channels and allocate their marketing budget accordingly.
Results:
☑️ 80% faster data processing for quicker insights and decisions.
☑️ Enhanced model precision through machine learning for optimized media spend and ROI.
☑️ 40% deeper model granularity for targeted resource allocation.
☑️ 5-week insights cycle for greater adaptability to market shifts.
☑️ $300 million in uncovered media buying opportunities for increased sales without additional spending.
AI Solution:
Seeking to address marketing challenges, the retailer implemented an AI-powered solution encompassing machine learning, natural language processing, and predictive analytics. This comprehensive AI suite transformed their marketing operations by automating data collection and analysis, enhancing model precision and granularity, shortening the insights generation cycle, and uncovering new media buying opportunities.
Key applications of the AI solution included:
● Automated data collection and analysis: AI algorithms streamlined the process of gathering and analyzing data from various sources, including customer transactions, website interactions, and social media engagement.
● Channel interdependency analysis: Machine learning techniques were employed to identify complex interdependencies between marketing channels, such as the impact of social media campaigns on e-commerce sales.
● Predictive analytics for demand forecasting: AI-powered models were developed to predict future customer demand and product trends.
● Personalized product recommendations: AI algorithms analyzed customer browsing behavior, purchase history, and demographic data to generate personalized product recommendations.
References:
1. How a leading US retailer used AI to improve marketing spend
Industry: Retail
Vendor: Inventiv Technologies
Client: Leading US Retailer
Previos Article Retail Giant Achieves 2x CTR, 3.2x Conversion Rate Gains with AI
Next Article 200% Reply Rate Surge with AI for Digital Marketing