AI Streamlines Agile Planning: Boosts Efficiency and Productivity
A I • May 24,2024
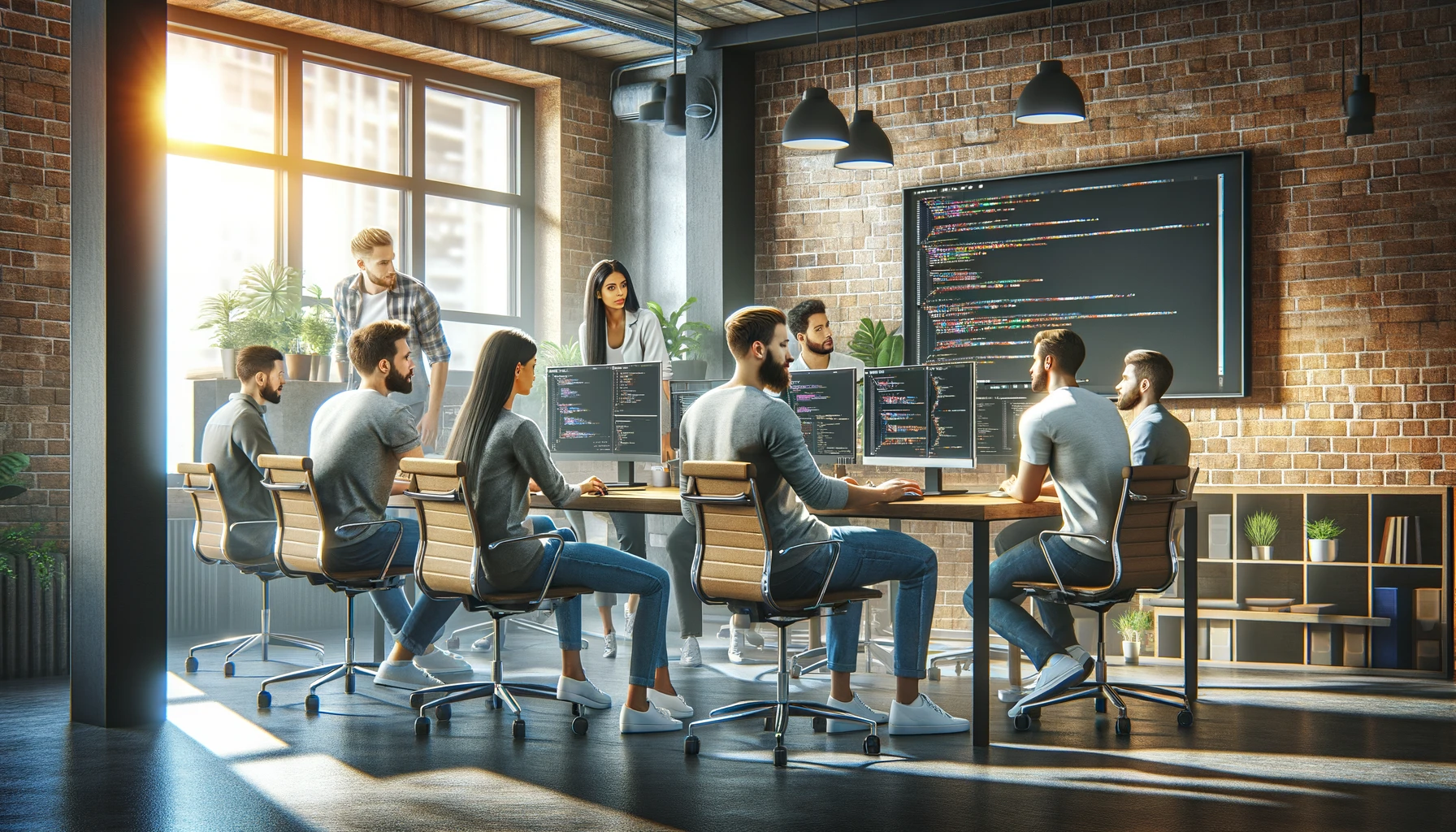
A software company struggling with inaccurate estimates and uneven workloads in agile sprints implemented an AI tool. This resulted in more precise planning, improved work balance, reduced risk of delays, and ultimately, higher quality deliverables on time.
Problem Statement:
A software company that adheres to an agile project management approach faced challenges with sprint planning. Inaccurate work estimates and uneven workload distribution hampered their process, leading to the following:
● Inefficient resource allocation: Unbalanced workloads could lead to burnout and hinder team performance.
● Missed deadlines: Inaccurate estimates increased the risk of delayed project completion.
● Suboptimal project execution: Inefficient planning could have a negative impact on project quality.
Results:
☑️ More Accurate Estimates: Task duration forecasts became more precise, leading to reduced variances and better planning. This translates to less wasted time and more efficient resource allocation.
☑️ Improved Work Balance: The AI tool optimized task distribution based on individual skills and preferences. This resulted in a fairer workload for each team member, boosting overall satisfaction and reducing the risk of burnout.
☑️ Reduced Risk of Delays: Early identification of potential delays through AI allowed the team to take proactive measures. This minimized disruptions and ensured deadlines were met more consistently.
☑️ Enhanced Project Performance: Optimized sprint planning led to improved quality of deliverables delivered on time. This indicates better overall project execution and efficient resource management.
AI Solution:
To address these issues, the company developed an innovative AI tool designed to:
○ Analyze Historical Data: The AI tool examined historical sprint data to identify trends and patterns in work efforts and identify areas for improvement.
○ Refine Estimates: Machine learning algorithms continuously improved the accuracy of effort estimates based on past performance.
○ Optimize Task Allocation: The tool recommended task allocation based on a combination of individual skills, experience, preferences, and current workload for a balanced team distribution.
○ Identify Potential Delays: Proactive risk assessments allowed the team to anticipate potential delays and take corrective measures before they impacted the project.
Implementation:
Project managers and scrum masters used the AI tool during sprint planning sessions. The tool analyzed previous sprint data and provided recommendations for task allocation, effort estimation, and risk identification. Project leads then reviewed these insights with other relevant factors to finalize the sprint plan.
Key takeaways: Integrating AI into a company’s agile methodologies showcased its potential to tackle project management challenges effectively. Implementing the AI tool refined project planning, boosting team satisfaction and productivity.
References:
1. Two case studies where AI enables process optimization
Industry: Software Development
Previos Article Software Company Eliminates 70% of Technical Issues with AI
Next Article AI Empowers Insurance to Achieve Underwriting Excellence