Implementing AI in EHR Rollout for a Major Hospital Network in the United States
V G • Oct 15,2024
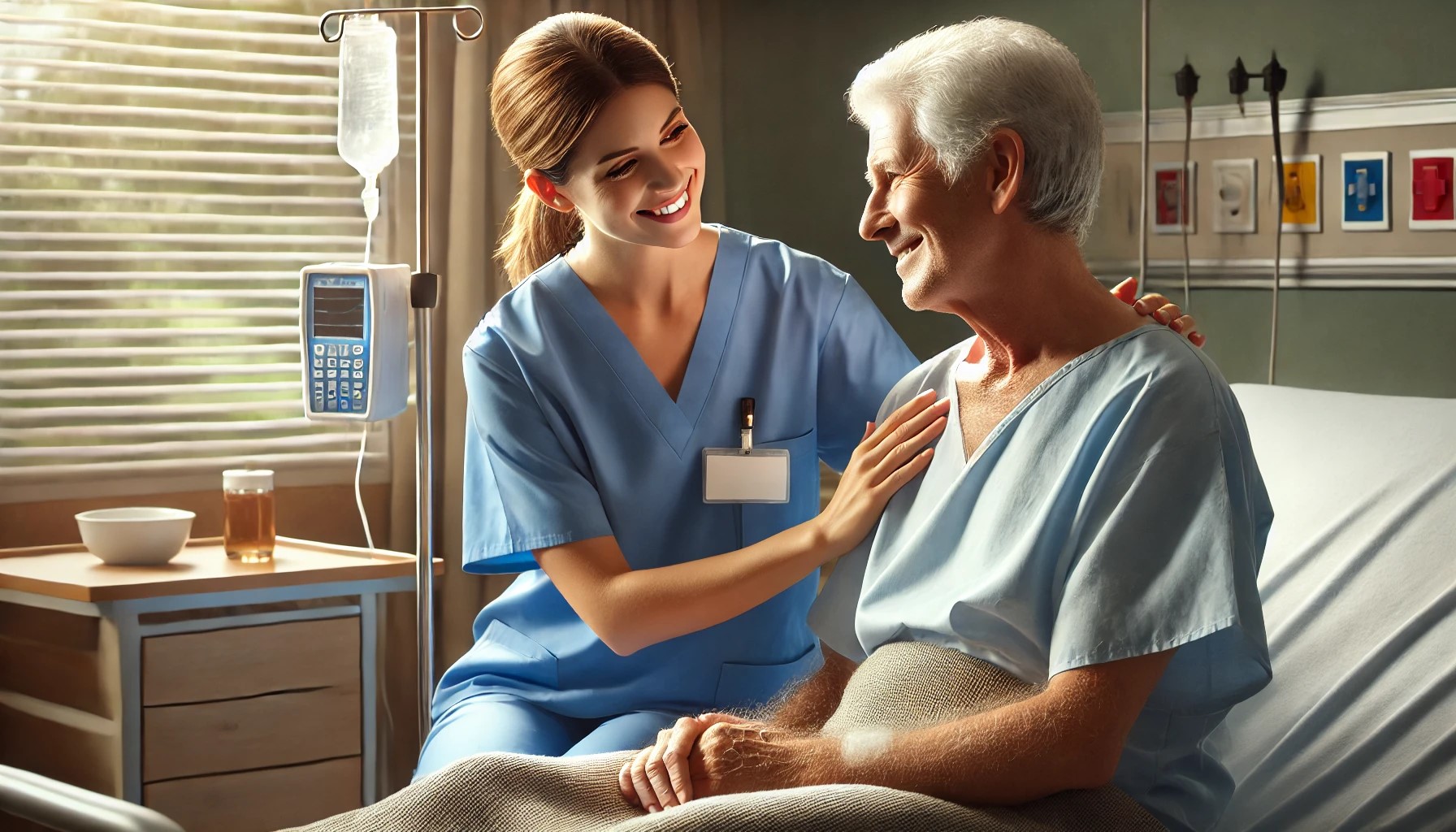
Summary:
By integrating AI into the EHR rollout, the major hospital network was able to overcome the challenges associated with data migration, interoperability, and user adoption.
Client:
Major hospital network in the United States
Problem Statement:
A major hospital network in the United States faced significant challenges in implementing a new Electronic Health Record (EHR) system across its facilities. The new EHR system aimed to standardize patient records, improve data accessibility, and streamline workflows for healthcare providers. However, the scale and complexity of the network posed considerable challenges, including managing data migration, ensuring interoperability with existing systems, training staff, and minimizing disruptions to patient care during the transition.
To address these challenges, the hospital network decided to integrate AI tools into the EHR rollout process. The AI implementation aimed to support data migration, improve user training, enhance system optimization, and ensure smooth workflow integration.
Results:
- Anticipated employee resistance and delivered specialized training programs.
- The EHR system was successfully and seamlessly integrated.
- AI oversaw adherence to healthcare regulations, minimizing the likelihood of legal complications.
AI Solution Overview:
The hospital network implemented an AI-based solution that addressed these challenges through several key functionalities:
- Automated Data Migration and Quality Assurance: The AI tool used natural language processing (NLP) to analyze unstructured data from the legacy systems, such as doctors’ notes and clinical summaries. This ensured that critical information was accurately mapped into the structured fields of the new EHR. Machine learning algorithms were deployed to identify and correct data inconsistencies, such as duplicate records and mismatches between patient demographics. This reduced the manual effort required for data cleaning and ensured the completeness of the transferred records.
- AI-Powered Interoperability Engine: The AI solution included an interoperability engine that used predictive analytics to map out data flows between the new EHR and existing medical devices and systems. This facilitated real-time data exchange, enabling clinicians to access lab results, imaging data, and vital signs directly through the EHR. The engine was designed to adapt to new data sources, making it easier for the hospital network to integrate future systems and technologies into the EHR environment.
- Personalized Training and User Support: The AI system analyzed usage patterns of the EHR by different user groups, such as doctors, nurses, and administrative staff, to identify common pain points and training needs. Using this data, it provided personalized training modules that targeted areas where users needed the most support. This adaptive training approach accelerated the learning curve and reduced user frustration.
- Workflow Optimization and Predictive Analytics: It provided predictive analytics to adjust staffing levels and allocate resources more effectively, ensuring that patient care was maintained even during periods of high demand. The AI also assisted in automating routine administrative tasks, such as appointment scheduling and follow-up reminders, freeing up time for clinical staff to focus on patient care.
References:
- AI in Project Management: Case Studies and Success Stories. https://www.zignuts.com/blog/ai-project-management-case-studies-success-stories
- AI in Project Management: Real-World Case Studies. https://www.linkedin.com/pulse/ai-project-management-real-world-case-studies-daun-hall-pmp-dasm-z6xtc/
- How AI is Transforming Healthcare: 12 Real-World Use Cases. https://medwave.io/2024/01/how-ai-is-transforming-healthcare-12-real-world-use-cases/
Industry: Healthcare
Client: A major hospital network in the United States
Publication Date: 2024