AI Enhances Quality Control for Pharmaceutical Water
A I • May 04,2024
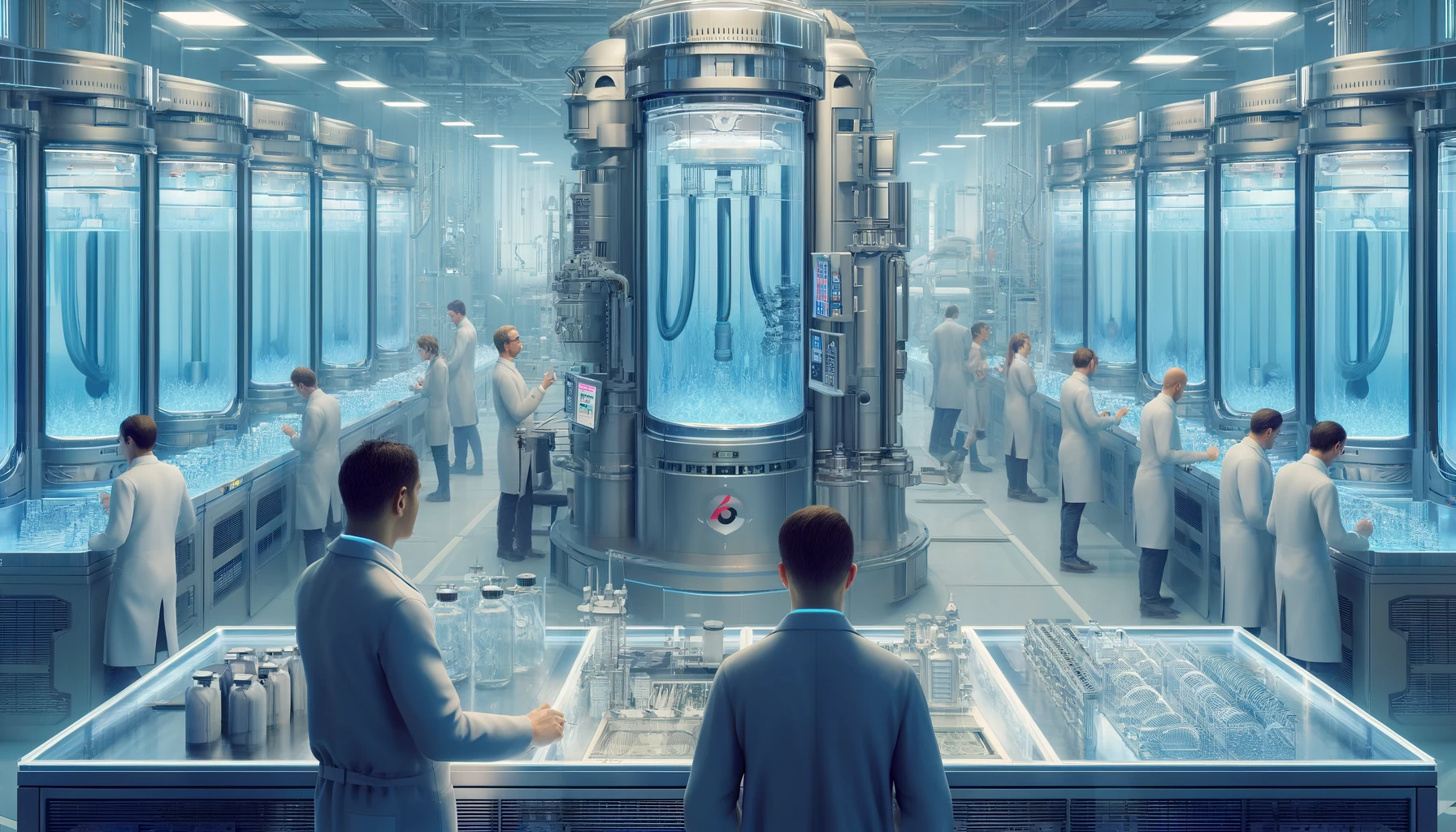
A pharmaceutical company struggled to meet the strict quality standards for water for injection (WFI) production. To address this issue, they implemented an AI-powered water purification system. This system significantly reduced water impurities, ensuring consistent compliance with pharmaceutical standards. The company also experienced a decrease in production costs and an improvement in overall efficiency.
Client:
A Water for Injection (WFI) plant supplies the pharmaceutical industry with highly purified water for injections. Stringent quality control is crucial for WFI plants. It ensures that the highest purity water is produced, which is essential for safe and effective medical products.
Problem Statement:
1. Annual plant shutdowns for preventive maintenance: This led to downtime and lost production.
2. Lack of a scientific approach to determining corrective maintenance needs: This resulted in some problems not being detected early enough and other issues being addressed sooner than necessary.
3. Manual data analysis: This was time-consuming and error-prone.
Results:
☑️ Reduced Downtime & Boosted Efficiency: AI-predicted corrective maintenance significantly reduced downtime, leading to higher production efficiency and output.
☑️ Lower Costs: The company’s adoption of AI led to decreased costs by minimizing downtime, enhancing production efficiency, and lessening the reliance on manual data analysis.
☑️ Enhanced Competitiveness: Cost cutting, in combination with enhancing efficiency, gives the company a competitive edge, attracting new customers and expanding its market share.
AI Solution:
To address the issue of traditional maintenance practices leading to unnecessary downtime and costs, an AI-driven predictive maintenance system was developed for WFI plants. This system utilizes machine learning (ML) algorithms to analyze sensor data, alarms, and water quality indicators to identify anomalies that could signal potential equipment problems.
Implementation Steps:
● Data Collection and Preprocessing: Sensor data, alarm records, and water quality metrics from 2018 were gathered. The data underwent cleaning, formatting, and transformation for analysis.
● Anomaly Detection: Machine learning algorithms were employed to examine the 2018 data and uncover patterns associated with past corrective maintenance events. This established a baseline for normal sensor readings and alarm behavior.
● Model Development: “Rule induction,” a machine learning technique, was utilized to create models capable of predicting anomalies based on sensor readings and alarm triggers. These models focused on identifying specific trends in the data preceding an anomaly (e.g., temperature or conductivity fluctuations).
● Model Testing on Historical Data (2018): The models were evaluated on the remaining 2018 data to assess their effectiveness in predicting anomalies. The “area under the curve” (AUC) metric was used to gauge the models’ performance.
● Model Testing on New Data (2020): Models trained on the 2018 dataset were applied to sensor data from the first half of 2020. The models successfully detected anomaly periods that coincided with actual maintenance events in 2020.
References:
1. Case Study: Water for Injection Plant AI-based Maintenance
2. Water for Injection Plant AI-based Maintenance
Industry: Water Treatment, Pharmaceutical
Vendor: Scientific research
Client: Water for Injection (WFI) plant
Keywords: AI for Quality Control, AI-powered Quality Management, AI for quality assurance (QA)
Previos Article AI Solution Drives 20% Defect Reduction for Food & Beverage Company
Next Article AI Strengthens Brewery Quality Control